Accelerating Early Drug Discovery with Open Protein-Small Molecule Binding Data
The SGC is embarking on a transformative phase to revolutionize drug hit discovery by enabling a shift from a largely lengthy experimental process to a fast-paced, data-driven, computational science. Our plan for the next five years is to create high-quality, openly accessible protein-ligand datasets, compatible with machine learning applications. By setting community challenges and focusing initially on hit-finding and hit optimization, we will benchmark machine learning approaches, with SGC hubs providing experimental testing of the predictions.
Our Open Data Strategy
SGC’s open data strategy is an ambitious and robust plan to spearhead the era of computational science in drug discovery. Using advanced technologies such as Affinity Selection Mass Spectrometry (AS-MS) and DNA-Encoded Libraries (DEL), along with innovative computational platforms like AIRCHECK, we are committed to data excellence and collaboration. This strategic approach positions SGC at the forefront of significant advancements in computational drug discovery and machine learning (ML) and artificial intelligence (AI) applications but also aligns with the Target 2035 initiative. By 2030, SGC and Target 2035 will have identified experimentally verified hits for thousand of human proteins and will push forward the development of open-access algorithms capable of predicting hits for proteins with no experimental data.
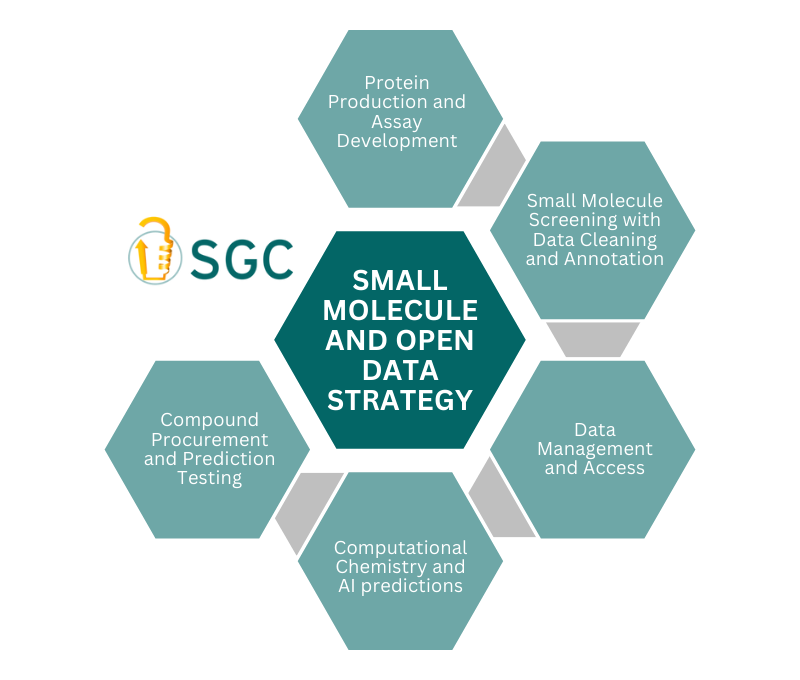
Why Generating Data is Needed
Transforming drug discovery into a computational process using ML/AI has the potential to significantly accelerate the identification of new therapeutic agents. However, a major hurdle is the lack of comprehensive, high-quality data to train sophisticated computational models. This shortage of data, combined with the need for greater data accessibility and empirical testing, severely limits AI's ability to make accurate predictions and accelerate drug discovery. SGC's open data strategy aims to bridge this gap by generating extensive, high-quality datasets that enable AI models and make small molecule drug discovery more accessible to all.
-
Affinity Selection Mass Spectrometry (AS-MS)
-
DNA-Encoded Libraries (DEL)
-
Benchmarking Purely Computational Approaches
Key Technologies
Affinity Selection Mass Spectrometry (AS-MS):
Our AS-MS platform is designed to generate large-scale protein-ligand interaction data. By screening thousands of different proteins, we will create an open dataset featuring over 50 million protein-ligand interactions. This initiative is supported by a 500,000-compound library, developed in collaboration with our Pharma partners.
DNA-Encoded Libraries (DEL):
We are leveraging the power of DNA-encoded libraries combined with machine learning. Key aspects include:
Public Domain Data: DEL screens for thousands of proteins will be made publicly available, allowing data scientists and ML/AI researchers to predict hits from commercial libraries.
Unprecedented Scale: We aim to generate a dataset exceeding 1 trillion data points, driving innovation and discovery.
Benchmarking Purely Computational Approaches:
We provide logistic and experimental support for the CACHE challenges; an initiative that benchmarks computational methods for small molecule discovery. The biophysics platform at SGC-Toronto acts as the experimental arm of CACHE, rigorously testing the predicted compounds and providing rapid, high-quality characterization of all the predicted hits. Our process involves screening predicted compounds, validating hits using orthogonal methods, and assessing their solubility and aggregation within a 3-month timeline. After this, we return the resulting data to participants for the hit-expansion round of each CACHE Challenge. Learn more about CACHE Challenges.
Open Data and AI Integration
We are currently working on making drug discovery more accessible by allowing researchers worldwide to use our high-quality datasets to speed up the development of new treatments:
AIRCHECK Platform: We are developing AIRCHECK, a data platform intended for sharing and analyzing AS-MS and DEL data. This rapidly growing cloud-based Artificial Intelligence-Ready CHEmiCal Knowledge base contains high-quality datasets of protein-small molecule binding data. Adhering to the FAIR (Findable, Accessible, Interoperable, and Reusable) principles, this valuable resource will soon be openly accessible to the scientific community.
- Protein and Data Hubs: Our experimental hubs generate reliable, high-confidence data. They will carry out biophysical assays and protein purification to test computational predictions and support the development of machine-learning algorithms.
How to participate
Join the SGC’s Open Data Strategy and contribute to our mission to democratize drug discovery and accelerate the development of new therapies.
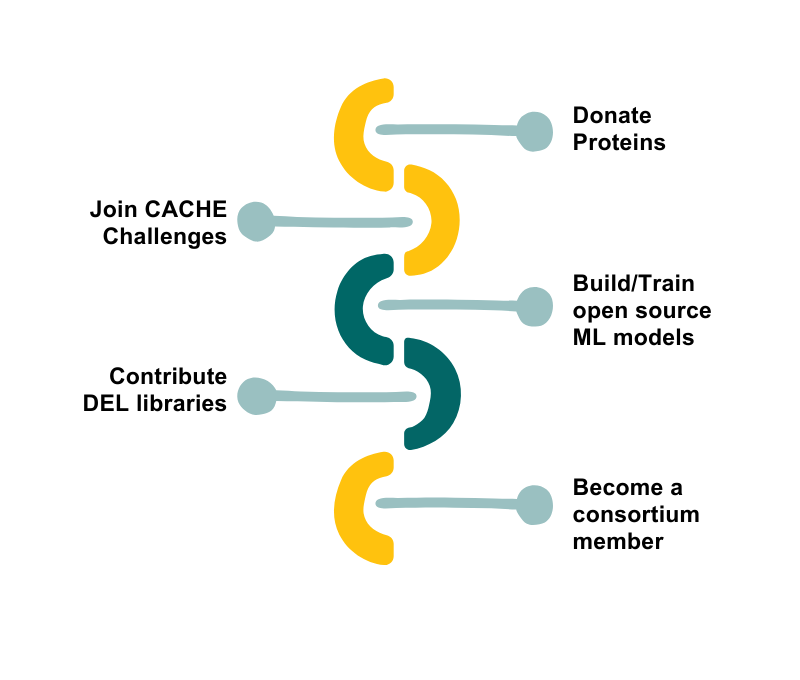